Finding the Stories Hidden in Data
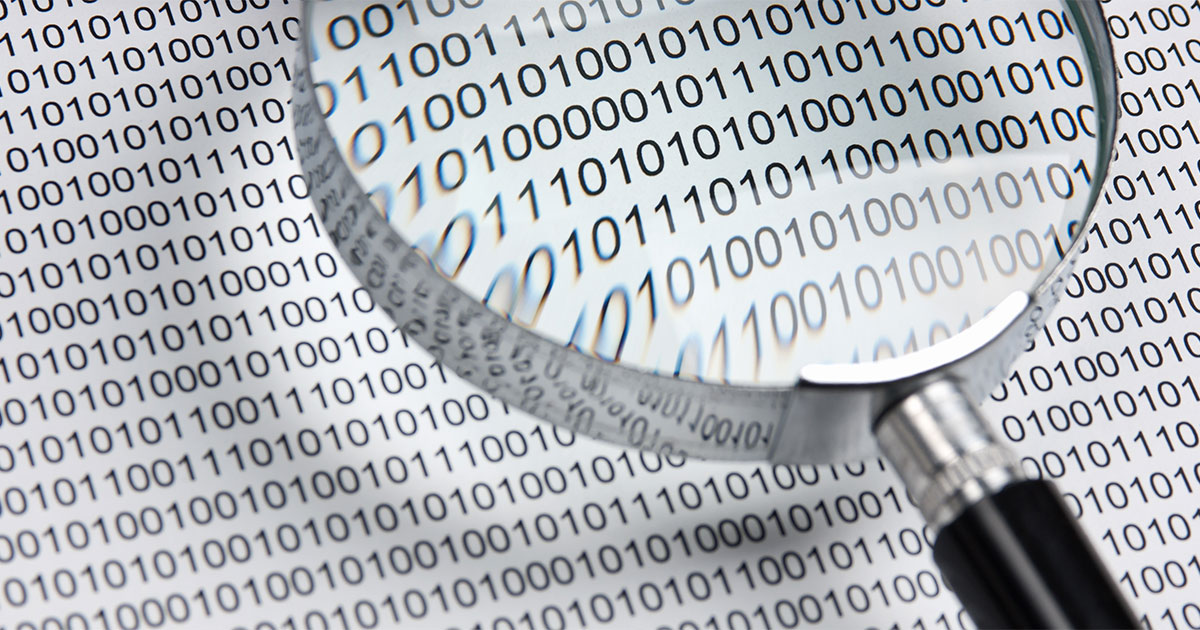
Babak Zafari is a data detective. Combing through multitudes of data, of bushels and bundles and buckets of numbers and information, he seeks the stories that are hidden there.
“Every data set has its own story,” says the assistant professor of statistics and analytics at Babson College.
In his research, Zafari often focuses on medical data, and what he tries to undercover there is the unusual and suspicious. “I find outliers,” he says. “I find extreme data points. I find anomalies.”
Those anomalies could very well be signs of fraud, waste, and abuse, which are big problems in the healthcare industry. Think of the physician who is improperly billing, or the patient who is visiting multiple doctors in order to obtain controlled substances.
Through his research, Zafari is able to model improved ways to find anomalies, which in turn helps medical auditors do their jobs better. “It is an area where I can apply my expertise and see the impact,” Zafari says.
Finding the Anomalies
So, how exactly can data help to find potential fraud? Zafari gives a simple example that, while not part of his research, can illustrate how that can work.
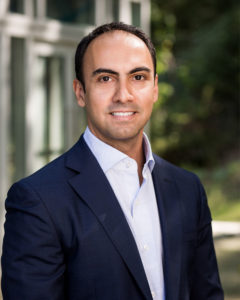
Consider, he says, how every medical claim has an estimated time associated with it. A regular office visit, for instance, may be expected to take 30 minutes. A tooth extraction, meanwhile, may have an estimated time of an hour. An investigator could look at all the claims a doctor filed in a year and then add up all the time those procedures should have taken. If the total time seems too high, as if the doctor might have had trouble completing all the medical procedures he claims to have done, that can raise a red flag.
“We don’t say that it’s necessarily wrong, but it needs to be investigated,” Zafari says, the suspicion being that the doctor was maybe making extra claims to receive the medical reimbursements associated with them.
Zafari clarifies that the job of modeling medical data is not to decide guilt or innocence. The healthcare industry is awash in data, from doctors, patients, and facilities, and that data is “not straightforward to handle,” Zafari says. The job of modeling, then, is to sift through this data and find those anomalies, those suspicious red flags, that warrant further investigating.
‘A Numbers Guy’
Zafari first fell in love with math in middle school. “I was always a numbers guy,” he says. As the years went on, he gravitated away from theoretical math, which concerns itself with the pillars and foundations of how equations work, and more to math with tangible uses. “Real-world application is the big part of it for me,” he says.
In our digital age, he’s now a statistician in a world overloaded with data, and the sheer amount of data available gives people like him a lot of raw material with which to work. “It can reveal patterns you didn’t know existed,” he says.
“The models are powerful, but if you push it too far, it will just confirm what you want to see. You want information that is meaningful and actionable.”
Babak Zafari, assistant professor of statistics and analytics
However, he does strike a note of caution, quoting the economist Ronald Coase: “If you torture the data long enough, it will confess to anything.” Elaborating, Zafari says, “The models are powerful, but if you push it too far, it will just confirm what you want to see. You want information that is meaningful and actionable.”
Furthermore, while there has been a big shift toward data-driven decision making, Zafari says that people should never be fully dependent on data. Intuition and expertise should still play a role.
Beyond Medicine
In his medical research, Zafari usually focuses on pharmaceutical data. To model it, he will use what is known as natural language processing. Designed to analyze text, natural language processing is the same technology that suggests words to you when you’re writing a sentence in an email, or determines whether the tone of a tweet is negative or positive.
For his research, Zafari will develop a model that looks at the text of prescriptions, finding the common billing patterns that exist in a particular medical specialty. For instance, he’ll look at cardiologists’ records and find the typical drugs that they prescribe. “We are recognizing patterns that exist in the data, then you find deviations in those patterns,” he says. “Once you know that average end point, you can compare it to every individual.”
Interestingly enough, Zafari also uses natural language processing to examine something a bit different than medicine: the entrepreneurial process. He is working on a research paper analyzing the conversation of teams at startup events, where strangers gather over the course of two or three days to hash out business ideas.
Looking at the words the team members use, Zafari can see which of them is taking more of a leadership role. Does the person who had the initial business idea stay in charge, for instance, or does someone else eventually take the reins of the venture?
The data gives Zafari a front-row seat to the entrepreneurial process, and he hopes to eventually publish his findings. “It will be a very interesting paper,” he says.
Posted in Insights